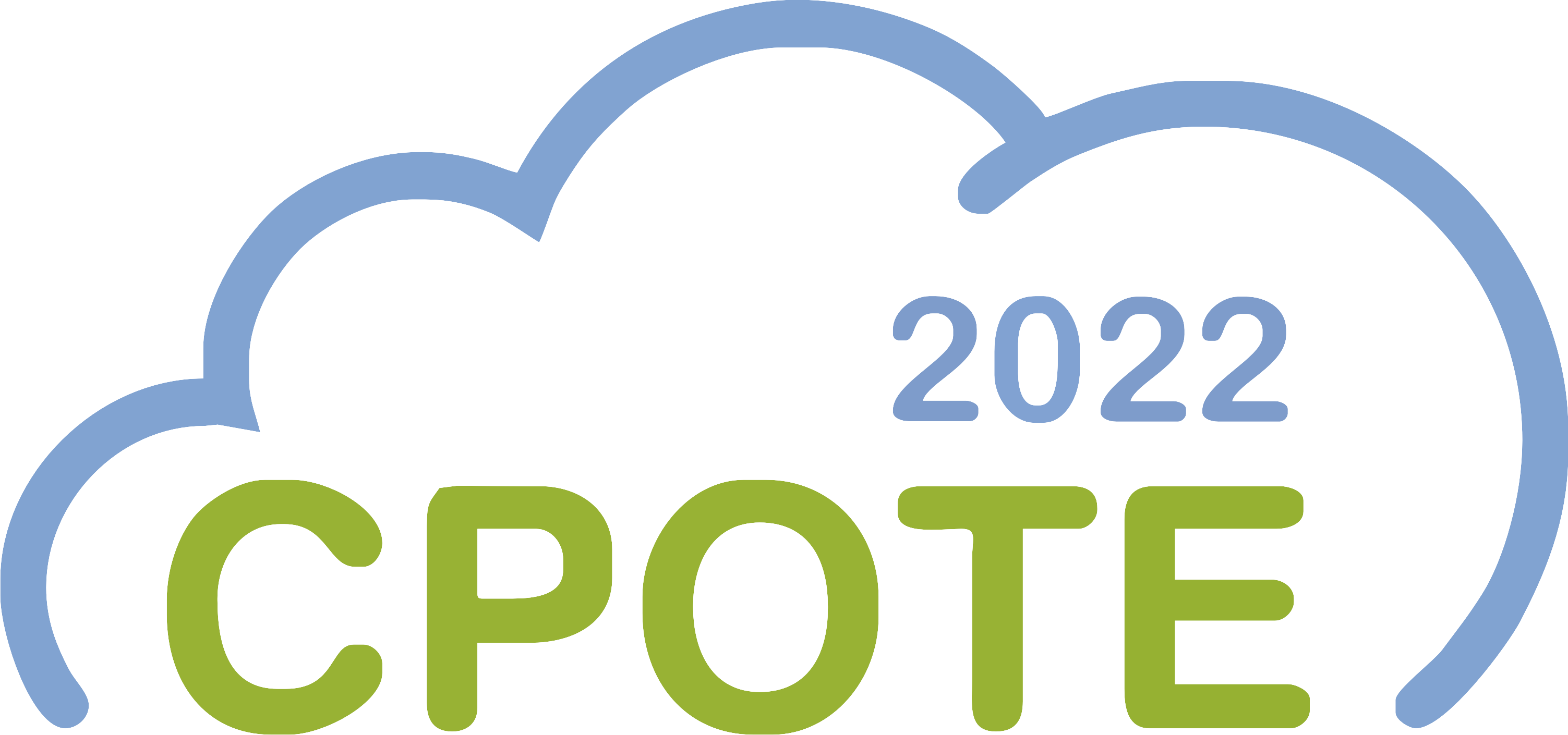
CPOTE2022
7th International Conference on
Contemporary Problems of Thermal Engineering
Hybrid event, Warsaw | 20-23 September 2022
7th International Conference on
Contemporary Problems of Thermal Engineering
Hybrid event, Warsaw | 20-23 September 2022
Abstract CPOTE2022-1161-A
Book of abstracts draft






Surrogate model for thermal storage tank heat losses estimation applied to concentrating solar power plants
Vinicius MORAIS, Federal University of Bahia, BrazilJulio SILVA, Federal University of Bahia, Brazil
The thermal energy storage system (TES) is the main feature that allows concentrating solar power plants (CSP) to accumulate thermal energy for electricity generation in the absence of solar radiation. Thus, the plant autonomy is directly related to TES design with respect to thermal capacity and heat losses calculations. This work presents the development of a surrogate model to predict storage tanks heat losses from input data related to ambient conditions, tank’s design, and its stored fluid (HTF). An analytical model is proposed and validated based on reference values of TES heat losses from real CSP plants found on the literature. A set of heat loss calculation data is carried out using the analytical model for surrogate training using MATLAB®’s machine learning toolbox. The results show that around 97% of the surrogate prediction is within a mean error of 650 W of heat loss predicted using the analytical code. Additionally, it was observed that computation time was reduced by 70% to calculate similar conditions of heat loss, which is beneficial for either extensive CSP plant simulation or design optimization routines.
Keywords: Surrogate modelling, Thermal storage systems, Heat loss estimation, Machine learning, Concentrated solar power plants (CSP)
Acknowledgment: The authors would like to thank the Grupo Global Participações em Energia (GPE) for their financial support under the Agência Nacional de Energia Elétrica (ANEEL) P&D program, project number PD-06961-0011/2019.